What would make a computer biased? Learning a language spoken by humans
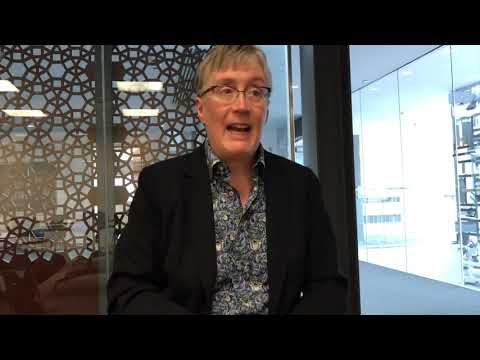
Researchers explain why artificial intelligence systems that learn a human language acquire the same gender and racial biases as the people who speak it.
- Share via
One of the amazing (and scary) things about artificial intelligence programs is that in learning to mimic their human masters so perfectly, these wonders of computer software hold up a mirror to patterns of behavior we engage in every day but may not even notice.
Beyond their extraordinary usefulness in industry, medicine and communications, these “learning” programs can lay bare the mental shortcuts we humans use to make sense of our world.
Indeed, new research with artificial intelligence programs highlights the ethnic and gender biases of English speakers.
In a first-of-its-kind effort, a group of Princeton University computer scientists set a widely used artificial intelligence program to the task of learning English by performing a massive “crawl” of the World Wide Web.
After gobbling up some 840 billion words, the software developed a vocabulary of 2.2 million distinct words and the fluency to use them in ways that were grammatically correct. It captured meaning and context by taking note of word associations — the regular proximity of certain words to certain other words.
In doing so, the program learned to anticipate that certain occupations, such as “computer programmer” and “doctor,” were more likely to be associated with masculine words like “boy” and “man.” The word “mathematics” also drew more associations to words suggesting male gender than it did to female gender.
And when it saw references to female names instead of male ones, the program was more likely to make associations to home and family words than to career words.
These findings, published this week in the journal Science, are in line with studies of “implicit bias.” In this burgeoning field, psychologists plumb people’s unconscious beliefs and biases by asking subjects to draw rapid word associations and timing the speed and frequency with which they make those associations. When words “feel” like they go together, the associations we make come readily (think “happy” and “puppy”). We’re a little less quick to draw a link between words we find incongruous (think “happy” and “criminal”).
Since the first pioneering work on implicit bias was published in 1998, researchers have found over and over again that Americans make assumptions, use stereotypes and carry bias along lines of gender, race and sexual orientation.
Despite apparent progress, our innermost beliefs do not always make a pretty picture.
Few of us explicitly own up to holding pejorative views toward African Americans, for instance, or to automatically assuming the gender of a doctor or a computer programmer. But as surely as it exists inside of virtually all of us, bias is enshrined in contemporary English language as well. That’s why the researchers’ artificial intelligence program was able to find it.
Along with its mastery of contemporary English, the program evidently picked up a few surprising attitudes as well: When it detected a name that “sounded” like that of an African American, it was less likely to conjure up pleasant associations (like the words “gift” or “happy”) than when it detected a typically European American name.
Of course, artificial intelligence programs only know what they’ve been taught (and despite some great film plots, they don’t actually believe anything). And lead author Aylin Caliskan, a computer scientist, acknowledges that her group’s findings leave a major question unanswered. Among them: whether the associations embraced by the AI software are responses to existing facts (such as the fact that women’s participation in the workforce still lags behind that of men), or whether they reflect the accumulated biases and beliefs that are baked into the English language itself.
“We don’t know yet” which it is, said Caliskan. After all, she added, “language contains facts about the world, and these facts can be cultural — about humans and their beliefs — or they can be statistical facts.”
Anthony G. Greenwald, the University of Washington social psychologist who pioneered the study of implicit bias, suggests that the new study should make us reconsider our assumptions about the impartiality of machines.
“In addition to revealing a new comprehension skill for machines, the work raises the specter that this machine ability may become an instrument of unintended discrimination based on gender, race, age, or ethnicity,” Greenwald wrote in a commentary that accompanies the study.
As learning programs play an ever-growing role in the conduct of modern affairs — diagnosing illnesses, winnowing job candidates, projecting the outcomes of complex decisions — the people who rely on them may face some of the same challenges we do when we confront our own biases. They may have to ask themselves whether their advice relies on reality or on the often-faulty assumptions we make about reality.
“Hopefully, the task of debiasing [artificial intelligence] judgments will be more tractable than the as-yet-unsolved task of debiasing human judgments,” Greenwald wrote.
ALSO
Type 2 diabetes, once considered a disease for adults, is increasingly common in tweens and teens
Archosaur fossils found in Tanzania are forcing scientists to rethink the evolution of dinosaurs
Even in choosing science books, Americans seem divided by politics